
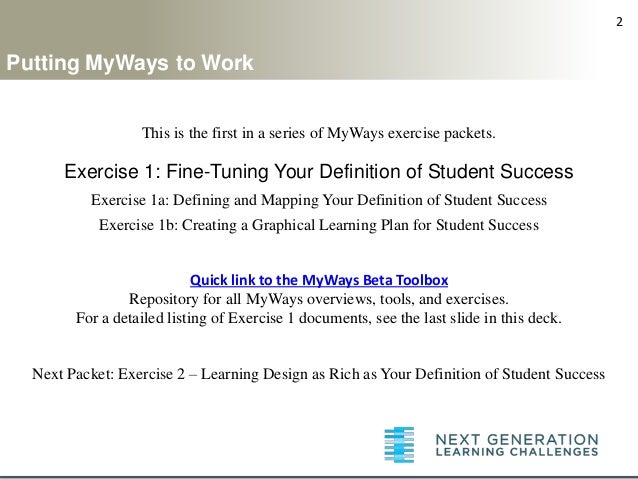
When carbon-free energy is produced on board, ships are less reliant on diesel-powered generators, which means less fuel is consumed by the vessel. Up to three HeatPower 300 Marine units can be installed on a single vessel, depending on the available thermal energy, delivering up to 1MW of renewable power. Its modular, scalable and SOLAS-compliant design ensures maximum onboard waste heat can be repurposed into clean electricity. Created for larger vessels with an engine size from 15MW and up, Climeon’s latest HeatPower product delivers superior economic and environmental benefits, alongside reduced payback times and streamlined installation. Developed for the maritime industry, HeatPower 300 Marine generates clean electricity from low-temp waste heat produced by a ship’s engines.Ĭapable of producing up to 355kW of carbon-free electricity from a single unit, HeatPower 300 Marine delivers enhanced performance and optimal energy output. G:\OneDrive\chath_curtin\OneDrive - Curtin\Research\dev\python\_installers\fastlogging-masterĬ:\Users\chath\AppData\Roaming\Python\Python39\site-packagesĬ:\Users\chath\AppData\Roaming\Python\Python39\site-packages\fastlogging-1.0.0-p圓.9-win-amd64.Climeon AB is launching its new waste heat recovery technology, HeatPower 300 Marine, on the maritime market at SMM, 6-9 September 2022. G:\OneDrive\chath_curtin\OneDrive - Curtin\Research\dev\python\DLN Sys.path : g:\OneDrive\chath_curtin\OneDrive - Curtin\Research\dev\python\DLN\tutorials\02_dl\01_pytorch\frameworks\01_fastai\fastai\Bugs\1 Learn = vision_learner(dls, resnet34, normalize=False, metrics=error_rate) # FIX: normalize=False = broadcast_vec(1, 4, an, self.std) # FIX: Move mean, std to GPUĭls = om_name_func(path, files, label_func, item_tfms=Resize(224),īatch_tfms=om_stats(,, cuda=False), # FIX: cuda=False, store in memory (do not move to GPU) = broadcast_vec(1, 4, an, self.std) # FIX: Move mean, std to GPU before normalizing x
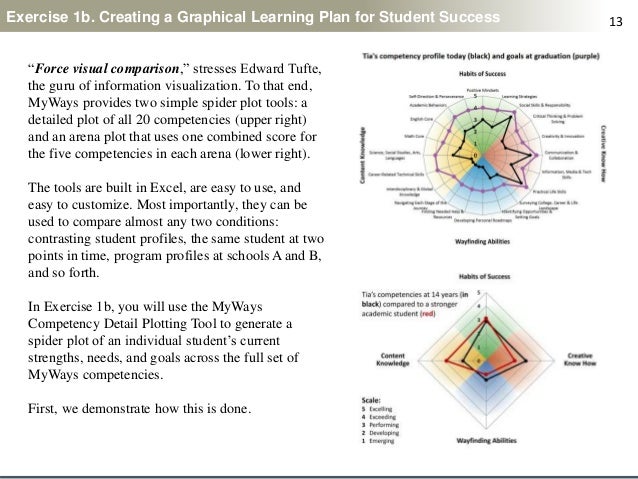
Return cls(*broadcast_vec(dim, ndim, mean, std, cuda=cuda)) # TfmdDL(DataLoader): to() method will put the `parameters` above, to GPU # FIX: (stop moving `parameters` to GPU)ĭef _init_(self, mean=None, std=None, from_stats(cls, mean, std, dim=1, ndim=4, cuda=True): Temporary Fix (highly inefficient): #!/usr/bin/env python _docs=dict(encodes="Normalize batch", decodes="Denormalize batch")

an,self.std = x.mean(self.axes, keepdim=True),x.std(self.axes, keepdim=True)+1e-7ĭef encodes(self, x:TensorImage): return (an) / self.std # <= mean, std here is 0į = to_cpu if x.device.type='cpu' else noop "Normalize/denorm batch of `TensorImage`"ĭef _init_(self, mean=None, std=None, axes=(0,2,3)): from_stats(cls, mean, std, dim=1, ndim=4, cuda=True): return cls(*broadcast_vec(dim, ndim, mean, std, cuda=cuda)) Ref: fastai-v2 Normalize Class class Normalize(DisplayedTransform): Your suggestions / ideas are highly appreciated. If anyone is familiar with or having the same issue, please let me know. Once I use my own Normalize2 class as shown below (keeping mean, std in the memory and moving them to GPU every time encodes is called - highly inefficient), it works.
#Finetunes meaning windows
This problem should have something to do with CUDA and accessing GPU tensors (mean, std) with multiple windows processes, according to my initial study. This is because initially, mean and std are moved to GPU when om_stats(…) is called, and by the time batches are formed from multiple processes and follow Normalize.encodes(…), the mean and std are taken as 0 in GPU. While feeding the batches from multiple processes (num_workers=1 or > 1), the batches are found to contain nan due to the division mean and std being 0 (ref Normalize.encodes) Resnet34 (pre-trained) model uses pre-trained weights hence vision_learner calls om_stats(…) with pre-trained resnet relevant mean, and std which will be moved to GPU (if cuda is available) Platform: Windows 10圆4, GPU: Nvidia 1080Ti Learn = vision_learner(dls, resnet34, metrics=error_rate) # normalize=True by default t_start_method('spawn') # default for windows Path = untar_data_ex(url, base=Path(ction('DATA_PATHS').FASTAI_DATA))ĭls = om_name_func(path, files, label_func, item_tfms=Resize(224), num_workers=1) # verbose=True Please refer to the program below: from import *įrom import untar_data_exįrom import EnvConfig
